Here are our four key takeaways, and a welcome update, that we took from the FIA IDX conference in London last month.
Leveraging Gen AI and Document Intelligence in Financial Services Operations
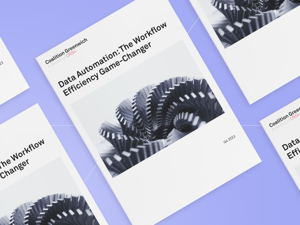
Data automation: the workflow efficiency game-changer
New Coalition Greenwich report highlights the urgent need for tech and automation in capital markets.
Transcript
0:06
Hi everyone, thanks so much for taking the time to register and join us today in this webinar. We're really looking forward to discussing the ways that we can leverage generative AI and document intelligence in your operations across financial services.
Maybe I'll just start by introducing myself a little bit. So my focus is primarily on Xceptor’s strategic AI initiative. A little bit about Xceptor and why we're focused on AI at the moment.
Xceptor, as you know, has provided a data automation platform and solutions to financial services for 20 years. Some of the things about financial services are fairly specific qualities, such as they have very complex ever-evolving data structures that require a lot of treatment and a lot of complexity. It also has a regulatory environment that's ever-evolving. And so, as a result, we've really focused our platform on those two things. Providing excellence in data automation and providing operational complexity. With that in mind, a couple of years ago we really started looking at AI in anger.
A number of our customers who had established AI programs came forward to us and said, hey look, you know we've built this AI/ML model in our AI program and we're struggling to create end-to-end automation with it. So, we have the component, but how about the end-to-end automation. Around about that time we started delivering a number of solutions for the banks where an AI model was embedded within our automation platform.
So, we took the experience from that and what we started building was our AI framework which adds UX and controls and really enhances the user journey for someonewho's looking to automate with machine learning or AI. This framework continued to be really popular.
What we now see is a technology landscape that's changing or evolving. So, we've been really focusing a lot more on generative AI and general document models. It turns out that it really speeds up the time to market and expands the number of use cases that you can solve and has actually made the setup process simpler.
We're really taking a strategic view and making general AI capabilities as standard into our platform. And that's why we want to talk to you about these topics today and get some feedback from the wider community. A big thank you first of all to Audrey Costabile who's joining us from Coalition Greenwich today. I'll let her introduce herself.
Thank you so much Gil, and welcome everybody to our talk today. As mentioned, my name is Audrey Costabile and I'm a Senior Analyst at Coalition Greenwich. Lately, we've been approached and speaking about advanced technology and many of our research projects. I tend to focus on data optimization, data efficiency, workflow efficiency and other topics. And of course, these have a great intersection with advanced technology.
Before we get started, I just want to go through really quick housekeeping items. We're going to have a few polling questions. I've got three of them today throughout the session. So, you'll definitely have a chance to weigh into the conversation. And as we get into our discussion today from the audience, I would ask you to please add any of your own questions to the Q&A chat, which I believe is on the bottom of your screen. We'll cover those when we get to Q&A at the end. So, without further ado, Gil, back over to you. The agenda is going to be fantastic. So, you know what we're really going to do is we're going to touch on some of our observations of how technology change is impacting financial services operations. And then we're going to talk about how some of the steps that we've seen that organizations can deploy in order to maximize their investment in AI.
We're sort of at the tip of the iceberg at Coalition Greenwich for doing our advanced technology work in this area. In fact, we've got three ongoing studies around just generative AI, which is probably not that difficult to imagine. Certainly, we've been hearing a lot lately about financial institutions that are embracing Gen AI. And that's really being driven by the promise of efficiency and effectiveness versus other technologies like machine learning.
5:02
So I want to level set a bit before we get into the evolution of things like machine learning and over to the Gen AI topic by showing you this data that we recently collected in the past year pertaining to the adoption of advanced technology. So, I thought what was interesting about this just by way of background is that we reached out to buy side professionals and ask them what their use was and their adoption of different types of advanced tech, in this case AI and ML.
And as you can see, we've done this across a spectrum of different fixed income products. This happened to be a fixed income study that we do annually. Now what's interesting is that if you look all the way to the left at full adoption, there are some very high numbers there, right. We see for instance US mortgage and mortgage bonds 63% - full adoption, municipal bonds 41% - full adoption, CLO's 44% - full adoption. So, it's kind of a little bit of a snarky slide if you will because these figures are clearly very hard to believe, right. And the reason I show this slide is not because I feel that's unreliable. I really think it speaks to how early in the process we are and where the understanding of what the use of AI and ML actually is and where it's heading.
I guess maybe a great place to start in light of these findings or non-findings if you will. Whatever way you want to look at, it will be interesting to see how these questions evolve over time.
I'd like to ask simply what is Gen AI and how does it actually differ from machine learning? Because I think there needs to be some more understanding about those specifics.
And we can talk about the experience that we've had working in the space. So, I've got a slide here that hopefully brings some of those topics out. First of all, on the left hand side - machine learning. I think probably that's been around for at least 10 years. You know what is it? So, it's quite in the technical approach to AI. So, it's often managed by an AI center of excellence populated by data scientists. And often what we're doing is creating a model, often based on one of the many technical analytical model types that you can start with and then it's trained. Often with just a few examples to get to a kind of a POC or a proof of concept. But then often there's a big hurdle where you're training on almost thousands of positive examples to get to a place where you have a production ready model.
Usually once that model's been trained, you're really pointing that model at one specific use. So, it usually has one or perhaps two use cases in the end and that’s quite important because as we move into the last couple of years where we've seen generative AI, and I think we're also including in this category general document models which behave quite similarly. But on the generative AI side, it's a little bit of a different ball game. So, you're obviously you're pre training the model on billions and billions of examples and that happens behind the scenes. And then it comes out-of-the-box ready to be instructed to carry out tasks by natural language and written text.
This is a massive advantage and actually can be considered different for operations in the sense that it now has very, very little technical requirements in terms of training it and setting it up. And it also comes out-of-the-box ready to solve like a whole different bunch of use cases. We've written some best practices on prompt writing or instruction writing. I guess it's kind of the new bit that people have to get to grips with. And we've likened it a little bit to working with a new intern, you know. So, the intern comes on the first day. They have very little to no context, but what you do is you provide them with instructions, and you provide them with as much context as you can. And they're ready and willing to carry out human activities and the more context you give them, the better it performs. And it's very, very similar to that in a way Audrey.
That's really interesting. We found also then speaking with our network there was some confusion about AI and ML and things that have been around quite some time, like rules-based methodologies, regression analysis. I think that's why some of the numbers in our findings were so artificially high and just people not having that clear understanding of what they were being asked and what the technology that underscored their systems. What was really relying upon.
9:58
I'd be curious where our audience is in this question in this particular adoption of advanced technology, and I'd love to pull up our first polling question now to kind of get a feel for that. As you can see you can type right into the interface of the platform. Are you presently using Gen AI in your role? There's a yes, there's no, no, but we plan to, or not sure. It's kind of a fair question as we've seen from our Coalition Greenwich results.
So those are coming in right now and what's nice is that we can actually see the live responses. I'm hoping that the audience can as well instead of those statistics as they come in. It looks like there's some forward-looking responses here with about a third saying they don't use it, but they plan to. About a third are hard no. And then of course, you have about a quarter of which are in the yes camp. And these are still kind of settling in right now. Relatively balanced, I think.
I think that matches quite well with what we're seeing as we're having conversations. There's a kind of hard core of earlier early adopters who've almost run away with a couple of use cases and started getting ahead of it. But what I would say is that most people that we talk to are at the moment trying to figure it out. And that seems to be the phase that is most popular from what we've seen. Well, maybe instead of focusing on where they are in the phase, something I think the audience might have been interested in is actually developing a better understanding of why the shift is actually happening.
What do you think is going on there? Yeah, I think people are seeing an opportunity. I think the most obvious thing that generative AI in general AI technologies have brought about is that it's reduced down the cost and the barrier to entry. And I think especially the upfront investment required to prove out a use case. I think with machine learning that was like a significant barrier that needed to be overcome and really you did a lot of work not knowing whether the end goal was actually going to be successful or not. And so it's had a really positive impact there. And I think as it's pulled down or it's improved the ROI, it means it's kind of pulled it down to a threshold where more use cases are coming into that kind of ROI threshold and so more becoming available.
I think secondly, you know just the non-technical nature of generative AI sort of combined with the fact that you can solve more use cases actually just fits a lot better with the operations transformation model for a number of reasons. But I think you know essentially, we've kind of been changed. We've kind of been pulled out of this era where the focus has been on how can I get the AI capability? But now we're moving into a new really exciting era where the focus is how can I take these general AI capabilities and apply them at scale but in a controlled way to important use cases? So, I think we're moving to that kind of application phase which is really cool.
Yes, for sure, it's interesting to see how different parts of the trade life cycle. So, you mentioned middle and back office you know that have been frustrated because of a number of different challenges right. And they can kind of get behind a technology that can be applied to so many use cases, particularly with some of the lower technical skills that might need to be enhanced with some of this tech. And I guess you know kind of changing gears a little bit, you know, maybe we can talk a bit about the steps taken to actually maximize investments in Gen AI. Because we talked about the shift to kind of getting there, what it is, but how are people actually selecting the use cases or the rapid iteration of use cases and, of course, you know with some emphasis on control too because that's been a large topic too. But maybe just start with how are they selecting use cases?
Yeah, absolutely. And we kind of taken the liberty here collating across our sales and presales teams and our implementation teams and try and pulling together the most common ones that keep coming up. So, you guys can take a snapshot of that. I think if you put generative AI use cases into Google you know what usually comes up is, assist AI, AI assisted developers writing code on autopilot, application copilots, like assistance for data retrieval, for an internal wiki. And I think all of these are pretty relevant topics really for banks.
15:01
But what we've really focused in on is automation of manual operation tasks. So it kind of surfaces itself as almost like a copilot for operations. And I think sometimes people would call this like autonomous automation agent or something to that effect, and it's not really any different to what we've been doing for years. But I think now you've got additional control efforts and priorities, but that you're seeing more use cases being sold for. So, I think on the slide we've just pulled out some of those like use cases first at the start.
I think document extraction is a really obvious one. We've been blown away by how out of the box it's able to capture that data at a really high accuracy which allowed us and others to move away from that kind of template per source traditional setup process. And it's especially powerful I think when you're dealing with full written prose in documents, and we can go into some examples in a bit. I think similar to that is contract analysis, so assistance making like legal decisions, reviewing actual legal documentation, comparing clauses, figuring out whether or not they're likely to be accepted is one of the ones that have really blown people away and has actually helped people manage risk.
I think routing and managing communication, I think that is part of that customer experience piece. But really being able to take communication that's coming inbound as part of an operational end-to-end workflow and take action on it straight away within a second without it ending up sitting in a queue for someone to get to, means that things like onboarding flows can be taken from days and weeks, can be taken to seconds and that really drives that kind of time to value. And finally, one really cool one is just on the side there, language translation.
We're writing a paper at the moment about GPT or ChatGPT 4’s effectiveness for translation. I think especially when financial services specific terminology is involved. And that again has been one of those kinds of standout use cases where people have kind of taken a step back and said OK, well, you know we were spending a lot of money actually on having this, this translated for ourselves and we're able to take that away.
Those are incredible use cases. And I just wanted to stop you for a second. I know people are probably taking notes and screenshotting, but I just want to make sure everyone is aware, you will have access to the slides after this as well. So as Gil is speaking about them, you don't have to do that. But I'm sorry, please continue.
I would probably say that there's still a number of scenarios where traditional machine learning works really well, especially if the subject matter is really specific or you need like a certain level of explainability. You're feeding in directly to a capital event or arbitrage or something. But often what we've found is like a combination of using rules with the right kind of orchestration techniques alongside general document models and alongside generative AI is how you then can turn those kind of user cases into those business cases that sit below.
So, in terms of the business cases, just moving down the page, what we've done is collated the top 4 in each sector across what the teams are working on and there's some really, really cool ones there. You know perhaps I'll pick a couple and I'll just talk a little bit about the, you know what's happening for each one. Loan notices is a really interesting one.
19:02
We see it so many times on the buy side where we see a lot of asset managers, hedge funds getting involved in the wholesale lending piece. Also, on fund admin side where we see the guys obviously outsourcing the work there. But often agent notices that are coming through are still sent by e-mail and instead of operations people spending time reading the e-mail, taking the attachment, figuring out whether it's a borrower or rate reset, having to take those fields and compare that to the internal system. Well, that can actually be done automatically and if you're at a higher level of confidence you can take all that out and actually book it into that core system STP. But otherwise, it's a much more efficient process just to be able to see the review of the AI assistant and then take a quick decision on whether to push it through.
The one really that's the standout or the most popular I guess across all the use cases that we've seen so far is, is number one underneath sell side and cap markets - OTC documentation. It's just enormously popular. I think the nature of OTC documentation is that it keeps getting more complicated as people search for new ways to achieve arbitrage. It's like the problem that everyone was hoping to solve but has actually just got worse. So, documents come in their confirmation documents, they have a combination of economics but then legal terminology that describes the trade because it's over the counter. So here you can massively reduce the risk by using like an AI assistant type automation to actually review the trade, review the confirmation. You can essentially go through the economics, you can actually go through the clauses, you can have the AI automation compare those clauses to an internal library and actually flag differences and actually suggest changes that would help you be able to accept or reject or come back with an update to that clause. I think that's been some of the most powerful demos and implementations that we've been able to offer.
You know what really strikes me about this slide is how extensive it is already, and we haven't been talking about generative AI for that long relative to other technologies. If you had to guess, how much more extensive could this slide or list become even in the next year or so? What is your thought on that?
We've cut the list at 4 so it already stretches, but I think this is what we keep telling our teams internally. We still don't actually know where the end of this thing is. Like what's the next big use case. I keep challenging our implementation teams, when you're in with the customers, try and work out how we can come up with that next big use case because it might be the next big thing that everyone's doing, you know for the next couple of years. I think you know really the sky is the limit.
Yeah, great points. We do have another polling question. This might be a good time to ask. This deals with the use cases that our audience is involved with for Gen AI. So maybe we can pull that up and you can see here that we're asking if you are using or what are the top use cases for Gen AI. Are you using it for document extraction at this time? For customer service, for communications management, contract manipulation, or something else? So, I'm very curious which of these categories is going to be most popular. But I see that document extraction is certainly bumping up rather quickly, which is not surprising, I don't think. But what do you think?
It definitely looks like document extraction is that kind of that everyone gets started with. Yeah, awesome. Yeah, that makes sense. We've even used it at Coalition Greenwich just to get through our earnings. I thought that was a very interesting use case for that just to get through these earnings statements. I guess like an awesome follow-up question here would be you know I don't think we have time unfortunately, but it's like to what extent are people using generative AI for document extraction by itself or combining it with other general document type technologies and those kinds of things.
OK, and so I think that we're probably kind of coming over to sort of the end of the topic of use cases but obviously related to use cases, is this notion of having to emphasize control. So, control is a huge AI topic. For example, we've seen that regulators are discussing what AI can and can't do given a certain amount of oversight and that's been ongoing. Maybe we could talk a little bit about what's happening there.
Absolutely so I guess one thing we didn't touch on in the previous section is that using generative AI, you’ve kind of short circuited that long ramp up to getting to production versions of an AI automation. And I think what that's really empowered is like a rapid iteration of use cases. So, if we've learned anything from the last 10 years is that digital transformation works best when the operations and the business are really closely looped into the development. And business and operations understand the use cases really well, so their feedback quickly iterates and then you end up with fantastic solutions.
So, the best way to achieve that really is by working in our hybrid model where it's sort of IT or at the center of it, concerned about control, but also model performance, model selection. But then they're able to empower people who are close to the business problem. And I think that kind of now creates this like new kind of focus on control as well. And it's like how do I control AI if it's going to be available for people who are kind of outside of the perhaps the IT world?
26:01
There's plenty of guidance coming down from regulators on how to think about AI. You've got the EU AI Act, you've got SR 11-7 and 10 and we've had some kind of guidance coming out in the UK from the Bank of England. But we're actually looking at, there's scheduled to be another legislation coming down on the 17th of May which will be really interesting. But I think generally from our partners and from customers, the interpretation is that there's an insistent that strong controls are put in place if AI is being used to arrive at a capital event.
So anytime a trade is being made or someone's life is being impacted, then you have to have really strong controls in place. And I think the sense among our customers is that you know a lot of the back office, middle office work that in the operations room and doesn't directly fall into this category. But nevertheless, the guidelines are probably going to be followed, you know out of respect for them. And so you know it's really important to have a really well defined policy. But then it's also important that all the technology that you're using helps you to make that a reality. And so that's why we've really, really been focusing on it in our Xceptor framework. I think the way we look at it, Audrey is in two parts. The first part is probably the biggest. So, it's operational control. To us this feels very reminiscent of the regulation coming out of 2008. You know if you think of BCBS 239 and the general regulations around control there, you know it was about putting in place the control, making sure that what you didn't have was business critical app processes being run or outside of enterprise application. Avoiding desktop apps, making sure you have a full audit, strict validation rules and in this case with AI having like strong confidence scores that find their way into a threshold which a human can review actions and operational oversight if they're needed in that space.
So, I think if we talk about generative AI and operational control, I think generative AI famously comes without confidence scores. But what you can do and what we've been working on is creating Gen AI evaluation workflows that allow you to kind of achieve something similar. And then so you can play that back into the control of the automation.
And I think you know from another sense; context of the business case really matters. I think one golden rule really is that the levels of control need to be proportionate to the level of determinism needed in the output. If you're running generative AI to suggest changes to a contract, you perhaps don't need to have so much control in place as if you're creating some kind of output that's going to be fed into a trading system.
You just mentioned automation and that's been a massive theme as well, obviously for some time now in all parts of the trade life cycle. We've seen it in our work and front office, middle office and certainly back office, particularly the automation of some of the more redundant tasks or tasks that don't require as much thinking so to speak. And just things that really lend themselves well to those types of processes. How would you say the integration of Gen AI applications is beneficial to automation? In other words, how does it enhance it? What should people be thinking about there?
30:12
We think general AI services has become, basically one of the technical components that Xceptor is built on. We offer Azure as our core AI service that Xceptor is built upon. And then drives a lot of the value from, but you can also plug in your own one. I think to support a new era of focus on rapid deployment of use cases that are powered by general AI capabilities, you need an automation platform that comes out-of-the-box ready to support the points that we've really discussed before. You need that kind of rapid iteration on use cases. Therefore, you need an automation platform that empowers the operations people and non-technical people to iterate over solutions.
And with this new focus on control, it's important that the automation platform that you embed your AI capabilities into also has out-of-the-box levels of control. And so Xceptor has been busy building this framework. I think what it does is it makes it easy to get those benefits from general AI capabilities safely into your workflows and your applications and your processes. I think some particular features that I think are important are having click to deploy user interfaces that empower non-technical people to iterate rapidly overuse cases. Having a strong orchestration layer that can seamlessly combine different things that can then enhance the automation.
I think the operational control framework which allows you to then build upon or combine multiple components. So generative AI, a general document model, business rules into one use case and then an integration layer because you really need to be able to hook into different systems in your environment to achieve that kind of full end to end. I think to get to value faster and save millions in getting AI properly baked into your processes, your applications, your workflows, your data pipelines, you need an automation platform that can help you there. And to keep AI and build AI into the daily lives of your teams.
That's great. I know that we're starting to get there towards the end of this discussion, and I'd like to bring up the topic that relates to getting started with generative AI. However, before I do so, I'd like to ask our audience one final polling question which relates to this topic. What we'd like to ask is how are you presently bringing in Gen AI models? How are you bringing them into production? Are you not doing so yet? Are you using automation software? Are you building your own pipeline? Or are you using desktop software?
As these results come in, and it's interesting that we're seeing many that are not doing so yet. Do you find that surprising Gil? Yeah, I think, I mean within that transition that we've seen, I think a lot of people have started off using machine learning models and are transitioning to generative AI yet. So, it doesn't necessarily surprise me too much there. I think it's really good to see that actually there are a few people already starting to use automation software. I don't think it's something that's kind of caught on just yet but is actually in the process of taking off. So that feels about right and its interesting stuff.
Great, and I'd like to remind everybody too since we are getting towards the end of the discussion, if you have any questions to please put them in the Q&A. I see we're already accumulating a lot of questions already. So that's great.
But anyway, turning back over to you, I mean, so how do we get started? I think, you know, we would love to continue the conversation. If you'd like to reach out to us here at the Xceptor team, we would love to spend a bit of time with you, talk about your use cases, fit them into things that we've perhaps seen or solved before and really get started.
At this point we've talked about the automation with Gen AI applications, I think you had spoken about AI itself, not necessarily being a differentiator. It's what solutions providers and you know the industry around us is actually doing with AI. Can you unpack that a little bit further?
35:20
With AI being so readily available on the scene, I suppose it's like OK, well how do you differentiate yourself? I think that's like an interesting point. I think it's important now about how you apply AI to use cases. I think it's less about having the AI because everyone's got it, but actually how you apply it to use cases. From Xceptor, we've done all the plumbing and the infrastructure and the compliance and user experience work to make Gen AI work for operations and that means you can just start using it for your use case and it's just baked in.
So that kind of ends up being a differentiator right there. But I think when you're thinking about automation platforms, it also matters what your differentiator was before the AI came along. So Xceptor, we focus in on financial services. We're always the tool for choice. If you've got a use case that has a lot of complex data structures that are evolving over time that happen all the time in financial services. And if you have a regulatory environment where your operational control is really important. Our differentiator hasn't changed, it's just that having the AI piece in there is really expanded the number of use cases we can solve and how easy it is to set up a lot of the requirements. So, I guess what I'm saying is it matters what your differentiator was before and how it's been enhanced.
Is there anything else you want to add, Gil, please do so. I think we're just about at the Q&A portion of our webinar and as mentioned, we've got many questions. So, thank you again everyone for submitting those. There's one question here that's very interesting and it asks how to think about whether or not AI is allowable or acceptable to be used in a business unit.
What type of review processes have you seen for potential AI enhancement?
So I guess we aren't with a vendor. We’ve basically gone through those processes ourselves. We've reviewed primarily Azure's AI services and they fit really nicely into the controls and processes that you need in a bank. We've been really happy with it, obviously provided by our hyper scaler. But I think each bank needs to basically address it in their own way, and our experience is that a lot of them already have already started looking at it. I think that perhaps that’s a question unfortunately you have to take internally to your organization.
This question, I was waiting for someone to ask this. If not, I was going to but what are the downfalls of Gen AI and machine learning?
I think, you know, one of the downfalls of Gen AI is that it is a little bit early. There are some cases where it kind of says the wrong answer and I think then a lot of that explainability is actually quite hidden, hidden behind the AI itself. I think there's still a lot of cases where machine learning works better in a case where you need to have really high levels of explainability or you need to be able to document in a really accurate way things like bias. I think it's fantastic and it solves a lot of use cases and can be deployed really quickly, but it just maybe isn't right for some use cases where there's a lot of scrutiny over the output in the end.
I know we're really coming down on time here but there is a question if you can give an example for instance - how do you actually integrate AI use case into the Xceptor workflow? Yeah so, so thanks for the question, Phil. I can see that one there.
We have built the Xceptor AI framework. We basically created a port that allows you to plug it in. It surfaces itself within our user interface and then you can configure how it responds through that. We've made it really, really straightforward case. We appreciate that a lot of banks have created their own machine learning, or their own AI programs and they want to be able to automate quickly with it. And so what we've done is we've made that port open so you can plug it in really, really fast.
40:17
For customers of Xceptor, especially the ones using our SAAS, then what we can do is bake in the AI. We use Azure Open AI Service and Azure's Document Intelligence so we can basically get you started really, really fast by shipping you Xceptor out-of-the-box ready to work.
Perfect, I know we have many other questions here and obviously these could take quite a bit of time. I'm suggesting that perhaps we end here because some of them are very specific, and Gil will reach out to you after the webinar. But I think at this point you, Gil, are there any final comments or thoughts you'd like to share with our audience before we do conclude?
So, there's one question here that maybe I'll just wrap up with. It's, are you considering Gen AI as a solution for co-piloting the Xceptor platform configuration? So yes, absolutely. That is on our road map. We're starting in very specific places. One of the challenges actually that customers raise a lot is taking a complex data structure and then mapping it to the structure that you want to achieve. And, at the moment, that's something that you can configure through the UI using drag and drop and some nice controls. But it is manual. What we're looking at, and this is kind of like a near term goal, is to have those kinds of exercises automated to a certain extent by a copilot. I think another layer to that is actually exposing our Xceptor documentation as a kind of chat layer, a copilot, in the user interface. That's not something that we're able to deliver in the near future, but it's something that we've certainly got our eyes on coming in the next number of years.
Fantastic, again, as you can see on this slide, there's an easy way to reach out to Xceptor if you have any additional questions and you'd like to speak with Gil. I'd just like to take a moment to thank everybody again for joining us today. And Gil, thank you, it's been a really interesting discussion.
Thank you so much, Audrey for spending time with us. It's awesome.
Explore our solutions
Capital Markets Operations
Our capital markets operations product supports automation and modernization of your post-trade operational processes.
Tax Processing
Our tax processing product enables end-to-end, automated, operational withholding tax processing capabilities.
Reconciliations
Helping financial institutions to automate simple to complex reconciliations end-to-end, providing greater data transparency, accuracy, and control.
Client Onboarding
Boost your ROI, guarantee compliance and minimize risks: discover how our platform can transform your client management and transaction workflow processes.
Get in touch
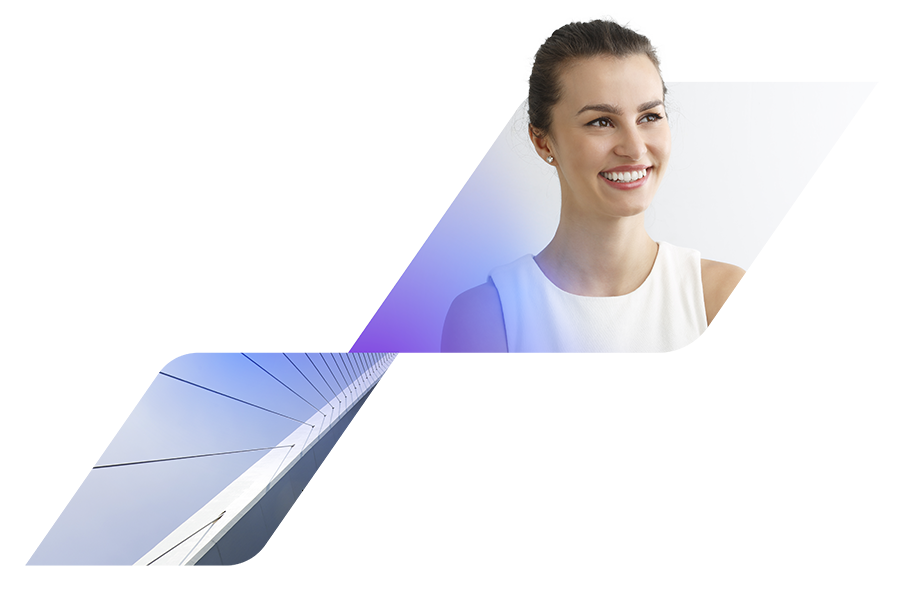
Featured insights and resources
Get our expert insights and resources to learn more about automating asset management processes, data management and how we’re supporting financial services transformation.